Understanding GraphCast, Google’s AI weather forecasting tool
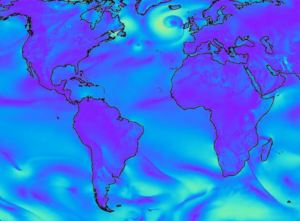
A research team at Google caused quite a stir in the weather world when it revealed that its new AI-driven “GraphCast” produces a more accurate forecast.
“They’re coming for our jobs,” one colleague wrote to me.
Not so fast.
Weather forecasting today and tomorrow
Traditional weather forecasts are produced by computer models that predict the future based on the current state of the atmosphere. These numerical weather predictions (NWP) use complicated physics and fluid dynamics equations to predict changes in the atmosphere from the ground up to the top of the troposphere. Programmers often tweak these models to improve the output, but they’re not perfect.
The forecast you see on your smartphone weather app comes directly from a forecast model. There’s no quality control. It’s raw data and it’s often wrong.
The forecast you get from your local television station and the National Weather Service is different. Meteorologists study the output of the models. Based on their understanding of local weather patterns and past experience, forecasters translate the data into a language the average person can understand. Even then, the weather sometimes turns out to be different than forecast.
Google’s GraphCast also uses computers to forecast the future. However, it doesn’t use sophisticated math and physics. Like local meteorologists, it relies on past experience. It predicts the future using historical weather data combined with machine learning-based weather prediction (MLWP.) In other words, artificial intelligence.
According to the project developers, GraphCast was trained using 39 years of archived data from the European Centre for Medium-Range Weather Forecasts (ECMWF). By comparing current weather patterns with similar weather patterns in the past, developers claim GraphCast can produce “an accurate 10-day forecast in under a minute.”
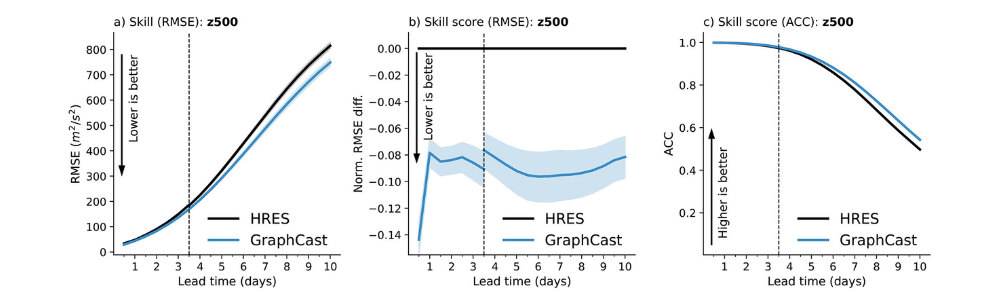
Like NWP, the GraphCast forecast process starts with the current state of the atmosphere. It also incorporates the weather conditions from six hours earlier. Based on these two inputs, as well as an understanding of historical weather patterns, the MLWP predicts the state of the atmosphere six hours into the future. Then using that forecast as the initial input, it predicts another six hours. And so on.
During testing, GraphCast outperformed the ECMWF’s High RESolution forecast (HRES) when comparing 1180 different parameters. Furthermore, although GraphCast is not specifically trained to predict tropical cyclones, atmospheric rivers, and extreme temperature changes, the forecasts were “generally superior” to the HRES.
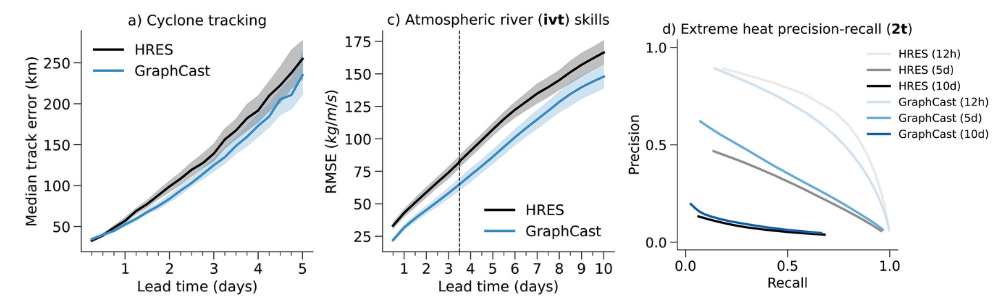
Read the full report here.
Past Performance Is Not Indicative Of Future Results
I can’t help but think of the disclaimer that financial advisors and mutual fund companies tell investors. Just because a stock went up in the past, doesn’t mean it’ll go up in the future. It might. But it might not.
Similarly, just because a major hurricane in the Gulf of Mexico made landfall near Houston in the past, doesn’t mean it will in the future. It might. But it might not.
“One key limitation of our approach is in how uncertainty is handled,” writes the development team. To that end, they don’t consider GraphCast a replacement for traditional numerical weather prediction. “Rather our work should be interpreted as evidence that MWLP is able to meet the challenges of real-world forecasting problems and has the potential to complement and improve the current best methods.”
The strengths of broadcast meteorologists
Broadcast meteorologists still possess unique strengths that allow them to compete with Google’s AI weather forecaster. One of the main advantages of human meteorologists is their ability to interpret and communicate weather information effectively to the general public.
People respond to other people. Looking at data on a weather app isn’t as helpful as having someone explain what that data means and how it might impact the local community. It’s the same reason, organizations hold press conferences in addition to issuing press releases. The message has more impact if you hear someone say it.
Broadcast meteorologists have the skills to convey complex weather forecasts in a way that is easily understandable to a wide audience. They can provide context, explain uncertainties, and offer insights based on their expertise and experience. Additionally, meteorologists can provide real-time updates during severe weather events, offering valuable guidance and reassurance to those affected.
The limitations of AI weather forecasts
Despite its impressive performance, GraphCast has certain limitations that broadcast meteorologists can leverage. One of the main challenges AI systems face is their dependence on observed weather conditions and historical data. GraphCast needs the ECMWF for initialization. And while AI technology might excel at analyzing existing patterns, it may struggle to forecast unprecedented or rapidly changing weather events.
Probably for that reason, developers say, “GraphCast can be retrained periodically with recent data, which in principle allows it to capture weather patterns that change over time, such as the effects of climate change, and long climate oscillations.”
Moreover, GraphCast and other AI weather forecasts lack intuition and contextual understanding. Weather patterns are not always straightforward. Meteorologists usually apply their knowledge and judgment to accurately predict complex situations. Additionally, broadcast meteorologists can provide localized forecasts and information for specific locations and events, which AI systems may struggle to replicate.
The importance of human touch in weather reporting
Weather reporting is not solely about data and predictions; it is also about connecting with the audience on a personal level. Broadcast meteorologists have the ability to establish a rapport with viewers, building trust and credibility over time. They can provide a human touch to weather reporting by sharing personal stories, explaining the significance of weather events, and addressing specific concerns of the viewers.
Furthermore, broadcast meteorologists can offer valuable insights beyond the numbers and charts. They can discuss the potential impacts of weather events on daily life, such as travel disruptions, outdoor activities, and safety precautions. This human element in weather reporting adds depth and relatability, making it a vital aspect that sets broadcast meteorologists apart from artificial intelligence.
Collaboration between broadcast meteorologists and AI technology
I look forward to the day when the Weather Workstation of the Future will help broadcast meteorologists monitor weather conditions, produce weather content, and distribute it on various channels and platforms.
Artificial intelligence can be a powerful tool to help broadcast meteorologists complete the daily workload. With the help of AI, broadcast meteorologists will have more time to analyze weather data, identify threats, and communicate potential impacts on their local communities.
READ MORE on TVNewsCheck: AI AND THE FUTURE OF BROADCAST METEOROLOGY
Tim Heller is an AMS Certified Broadcast Meteorologist, Talent Coach, and Weather Content Consultant. He helps broadcast meteorologists uplevel their performance and productivity by communicating more effectively and efficiently on-air, online, and on social media.